生物信息學(xué)習(xí)的正確姿勢(shì) ATAC-seq即transposase-accessible chromatin using sequencing,是一種檢測(cè)染色體開(kāi)放區(qū)域的技術(shù)。該方法由斯坦福大學(xué)Greenleaf實(shí)驗(yàn)室在2013年首次發(fā)表[1],兩年后該實(shí)驗(yàn)室又發(fā)表基于單細(xì)胞的ATAC-seq技術(shù)[2]。ATAC-seq相比于之前的FaiRE-seq和DNase-seq,ATAC-seq用Tn5轉(zhuǎn)座酶對(duì)染色體開(kāi)放區(qū)域進(jìn)行剪切,并加上adaptors序列(圖1的紅藍(lán)片段)。最后得到的DNA片段,包括了開(kāi)放區(qū)域的剪切片段,以及橫跨一個(gè)或多個(gè)核小體的長(zhǎng)片段。
 圖1. ATAC-seq[1]
根據(jù)片段長(zhǎng)度可以分為Fragments in nucleosome-free regions(<147 base pairs)和Fragments flanking a single nucleosome (147~294 base pairs), 以及更長(zhǎng)的多核片段。片段長(zhǎng)度分布如下圖,沒(méi)有跨越核小體的小片段最多,其次是單核片段,依次遞減。 圖2. DNA片段長(zhǎng)度分布 scATAC-seq建庫(kù)原理以10x 建庫(kù)方法為例[5],比較scATAC-seq 和scRNA-seq建庫(kù)方法的異同。 二者都用膠珠(GEMs)的方法,不一樣的是ATAC膠珠上的序列中不用UMI,因?yàn)榛蚪M只有一對(duì)序列,無(wú)需像RNA一樣定量。另外序列末端用接頭引物Read 1N代替PolyT。
 scRNA-seq通過(guò)結(jié)合cDNA的PolyA尾進(jìn)行擴(kuò)增,而scATAC-seq的DNA片段沒(méi)有PolyA尾,取而代之的是Tn5酶轉(zhuǎn)座剪切時(shí)插入的adaptors片段,可以與膠珠上的Read 1N序列互補(bǔ)。  DNA片段接上膠珠后,在另一端加Read2和Sample index序列。在此之前,scRNA-seq需要將cDNA酶切至合適的片段長(zhǎng)度,而scATAC-seq的片段不進(jìn)行打碎,接上Sample index和P7序列后進(jìn)行擴(kuò)增。
 最后上機(jī)測(cè)序。scRNAseq如果是3‘單端測(cè)序,Read2讀取最近的100bp讀長(zhǎng),而Read1只讀取16bp的細(xì)胞barcode序列和10bp的UMI序列,共26bp。scATAC-seq則用雙末端測(cè)序,讀長(zhǎng)一般不低于45bp[3]。

scATAC-seq最后可以得到4個(gè)原始文件:  其中I1/2分別是barcode和sample index,R1/2是目的片段的雙末端。
10x提供cellranger軟件對(duì)原始數(shù)據(jù)進(jìn)行初步分析,如質(zhì)控,比對(duì),peak calling等。 $ cellranger-atac count --id=sample345 \ --reference=/opt/refdata-cellranger-atac-GRCh38-1.2.0 \ --fastqs=/home/jdoe/runs/HAWT7ADXX/outs/fastq_path \ --sample=mysample \ --localcores=8 \ --localmem=64
質(zhì)控方法重要指標(biāo): Fraction of fragments overlapping any targeted region 覆蓋注釋區(qū)域的片段比例。目標(biāo)區(qū)域包括TSS,DNase HS,增強(qiáng)子和啟動(dòng)子等。一般要求大于55%。 Fraction of transposition events in peaks in cell barcodes 轉(zhuǎn)座位點(diǎn)位于peaks區(qū)域的比例。一般大于25%。如果比例過(guò)小,有可能是細(xì)胞狀態(tài)不好,或者測(cè)序深度不夠。 Enrichment score of transcription start sites(TSS) 轉(zhuǎn)錄起始位點(diǎn)的標(biāo)準(zhǔn)化分?jǐn)?shù)。閾值選擇根據(jù)基因組而定。人類一般選擇TSS分?jǐn)?shù)大于5或者6的細(xì)胞樣本[3]。如果分?jǐn)?shù)太低說(shuō)明細(xì)胞染色質(zhì)結(jié)構(gòu)瓦解,或者實(shí)驗(yàn)過(guò)程細(xì)胞裂解方式不當(dāng)。
 TSS標(biāo)準(zhǔn)化分?jǐn)?shù)的計(jì)算方法不同,可能導(dǎo)致閾值偏差。10X的標(biāo)準(zhǔn)化方法是cut sites數(shù)除以最小值,而ENCODE的標(biāo)準(zhǔn)是在cut sites數(shù)除以兩個(gè)末端各100bp的cut site數(shù)的平均值。由于一般越靠近中間數(shù)值越大,ENCODE的標(biāo)準(zhǔn)化分?jǐn)?shù)整體比10x的分?jǐn)?shù)小一些。

其他指標(biāo): - Fraction of read pairs with a valid barcode > 75%;
- Q30 bases in barcode > 65%;
- Q30 bases in Sample Index > 90%;
- Estimated Number of Cells 500~10000 +- 20%;
- Median fragments per cell barcode > 500;
- Fragments in nucleosomefree regions >55%;
- Fraction of total read pairs mapped confidently to genome (>30 mapq) >80%;
- Fraction of total read pairs in mitochondria and in cell barcodes < 40%;
下游分析(以Signac為例)Signac包由Seurat同一團(tuán)隊(duì)開(kāi)發(fā),獨(dú)立于Seurat包,在2020年8月開(kāi)始發(fā)布在GitHub上。目前仍是1.0.0版本[4]。 我們可以用10x官網(wǎng)的PBMC數(shù)據(jù)做演示。 首先加載所需R包: library(Signac) library(Seurat) library(GenomeInfoDb) library(EnsDb.Hsapiens.v75) library(ggplot2) library(patchwork) set.seed(1234)
加載peaks, 細(xì)胞注釋和片段分布數(shù)據(jù),并創(chuàng)建object。這個(gè)object和Seurat object類似,只是在assay里多了peaks等信息。 counts<-Read10X_h5(filename= 'atac_v1_pbmc_10k_filtered_peak_bc_matrix.h5') metadata <- read.csv( file = 'atac_v1_pbmc_10k_singlecell.csv', header = TRUE, row.names = 1 ) chrom_assay <- CreateChromatinAssay( counts = counts, sep = c(':', '-'), genome = 'hg19', fragments = 'atac_v1_pbmc_10k_fragments.tsv.gz', min.cells = 10, min.features = 200 ) pbmc <- CreateSeuratObject( counts = chrom_assay, assay = 'peaks', meta.data = metadata )
總共8728個(gè)細(xì)胞,87405個(gè)features。features不是基因,是基因組的注釋區(qū)域,如啟動(dòng)子,增強(qiáng)子等。 pbmc[['peaks']] ## ChromatinAssay data with 87405 features for 8728 cells ## Variable features: 0 ## Genome: hg19 ## Annotation present: FALSE ## Motifs present: FALSE ## Fragment files: 1
加載注釋: extract gene annotations from EnsDb annotations <- GetGRangesFromEnsDb(ensdb = EnsDb.Hsapiens.v75) # change to UCSC style since the data was mapped to hg19 seqlevelsStyle(annotations) <- 'UCSC' genome(annotations) <- 'hg19' # add the gene information to the object Annotation(pbmc) <- annotations
TSS和blacklist比例計(jì)算。 # compute nucleosome signal score per cell pbmc <- NucleosomeSignal(object = pbmc) # compute TSS enrichment score per cell pbmc <- TSSEnrichment(object = pbmc, fast = FALSE) # add blacklist ratio and fraction of reads in peaks pbmc$pct_reads_in_peaks <- pbmc$peak_region_fragments / pbmc$passed_filters * 100 pbmc$blacklist_ratio <- pbmc$blacklist_region_fragments / pbmc$peak_region_fragments pbmc$high.tss <- ifelse(pbmc$TSS.enrichment > 2, 'High', 'Low') TSSPlot(pbmc, group.by = 'high.tss') + NoLegend()

質(zhì)控。Signac包用5個(gè)指標(biāo)做過(guò)濾: TSS富集分?jǐn)?shù),大于2; blacklist比例,小于0.05; 纏繞核小體的片段與非核小體片段(< 147bp)的比例,小于4; 匹配peaks區(qū)域的片段比例,大于15%; 匹配peaks區(qū)域的片段數(shù),大于3000,小于20000。 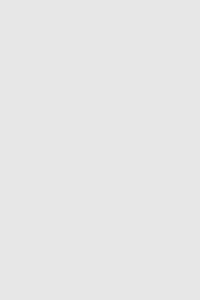
pbmc <- subset( x = pbmc, subset = peak_region_fragments > 3000 & peak_region_fragments < 20000 & pct_reads_in_peaks > 15 & blacklist_ratio < 0.05 & nucleosome_signal < 4 & TSS.enrichment > 2 ) pbmc ## An object of class Seurat ## 87405 features across 7060 samples within 1 assay ## Active assay: peaks (87405 features, 0 variable features)
降維聚類。 pbmc <- RunTFIDF(pbmc) pbmc <- FindTopFeatures(pbmc, min.cutoff = 'q0') pbmc <- RunSVD(pbmc) pbmc <- RunUMAP(object = pbmc, reduction = 'lsi', dims = 2:30) pbmc <- FindNeighbors(object = pbmc, reduction = 'lsi', dims = 2:30) pbmc <- FindClusters(object = pbmc, verbose = FALSE, algorithm = 3) DimPlot(object = pbmc, label = TRUE) + NoLegend()
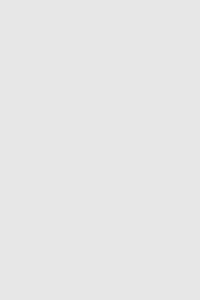 創(chuàng)建基因活性矩陣。之前的聚類區(qū)域所用的features是peaks,為了展示不同分群基因活性的差異,首先要?jiǎng)?chuàng)建一個(gè)類似RNA表達(dá)的矩陣。用基因加上游2000bp區(qū)域的比對(duì)片段數(shù)代表該基因的活性。
gene.activities <- GeneActivity(pbmc) # add the gene activity matrix to the Seurat object as a new assay and normalize it pbmc[['RNA']] <- CreateAssayObject(counts = gene.activities) pbmc <- NormalizeData( object = pbmc, assay = 'RNA', normalization.method = 'LogNormalize', scale.factor = median(pbmc$nCount_RNA) )
展示marker基因活性: DefaultAssay(pbmc) <- 'RNA'
FeaturePlot( object = pbmc, features = c('MS4A1', 'CD3D', 'LEF1', 'NKG7', 'TREM1', 'LYZ'), pt.size = 0.1, max.cutoff = 'q95', ncol = 3 )
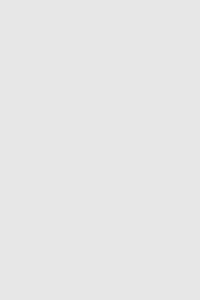 與scRNA-seq數(shù)據(jù)的整合分析。單細(xì)胞轉(zhuǎn)錄組數(shù)據(jù)地址
# Load the pre-processed scRNA-seq data for PBMCs pbmc_rna <- readRDS('/home/stuartt/github/chrom/vignette_data/pbmc_10k_v3.rds') transfer.anchors <- FindTransferAnchors( reference = pbmc_rna, query = pbmc, reduction = 'cca' ) predicted.labels <- TransferData( anchorset = transfer.anchors, refdata = pbmc_rna$celltype, weight.reduction = pbmc[['lsi']], dims = 2:30 ) pbmc <- AddMetaData(object = pbmc, metadata = predicted.labels)
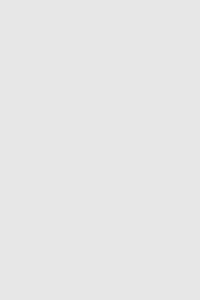 尋找細(xì)胞分群特異的peaks。
# change back to working with peaks instead of gene activities DefaultAssay(pbmc) <- 'peaks' da_peaks <- FindMarkers( object = pbmc, ident.1 = 'CD4 Naive', ident.2 = 'CD14 Mono', min.pct = 0.2, test.use = 'LR', latent.vars = 'peak_region_fragments' ) plot1 <- VlnPlot( object = pbmc, features = rownames(da_peaks)[1], pt.size = 0.1, idents = c('CD4 Memory','CD14 Mono') ) plot2 <- FeaturePlot( object = pbmc, features = rownames(da_peaks)[1], pt.size = 0.1 ) plot1 | plot2
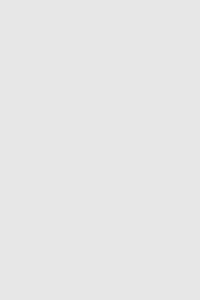 展示基因在不同細(xì)胞類型的開(kāi)放程度:
# set plotting order levels(pbmc) <- c('CD4 Naive','CD4 Memory','CD8 Naive','CD8 Effector','DN T','NK CD56bright','NK CD56Dim','pre-B','pro-B','pDC','DC','CD14 Mono','CD16 Mono')
CoveragePlot( object = pbmc, region = rownames(da_peaks)[1], extend.upstream = 40000, extend.downstream = 20000 )
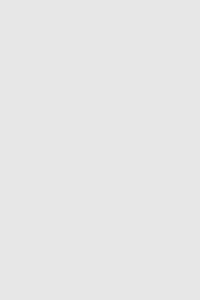 此外還有其他分析,如TF footprinting等。footprinting顧名思義是指轉(zhuǎn)錄因子留下的印記,由于Tn5酶不能剪切到TF結(jié)合的區(qū)域,所以footprinting圖相對(duì)與TSS圖,中間有“凹陷”,凹陷的程度根據(jù)TF結(jié)合的時(shí)間確定[6]。
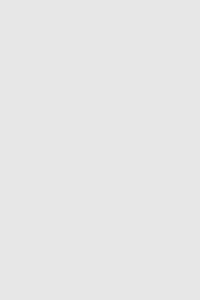
總的來(lái)說(shuō),Signac包是一個(gè)親民實(shí)用的工具。雖然有一些不足的地方,如染色體的注釋目前只能選擇UCSC的,不能選Ensemble。 參考文獻(xiàn):
[1] Buenrostro, J., Giresi, P., Zaba, L. et al. Transposition of native chromatin for fast and sensitive epigenomic profiling of open chromatin, DNA-binding proteins and nucleosome position. Nat Methods 10, 1213–1218 (2013). https:///10.1038/nmeth.2688 [2] Buenrostro, J., Wu, B., Litzenburger, U. et al. Single-cell chromatin accessibility reveals principles of regulatory variation. Nature 523, 486–490 (2015). https:///10.1038/nature14590 [3] https://www./atac-seq/ [4] https:///signac/index.html [5]https://assets./an68im79xiti/Cts31zFxXFXVwJ1lzU3Pc/fe66343ffd3039de73ecee6a1a6f5b7b/CG000202_TechnicalNote_InterpretingCellRangerATACWebSummaryFiles_Rev-A.pdf [6] Sung, M., Baek, S. & Hager, G. Genome-wide footprinting: ready for prime time?. Nat Methods 13, 222–228 (2016). https:///10.1038/nmeth.3766
|